Understanding the Importance of Machine Learning Data Labeling in Business
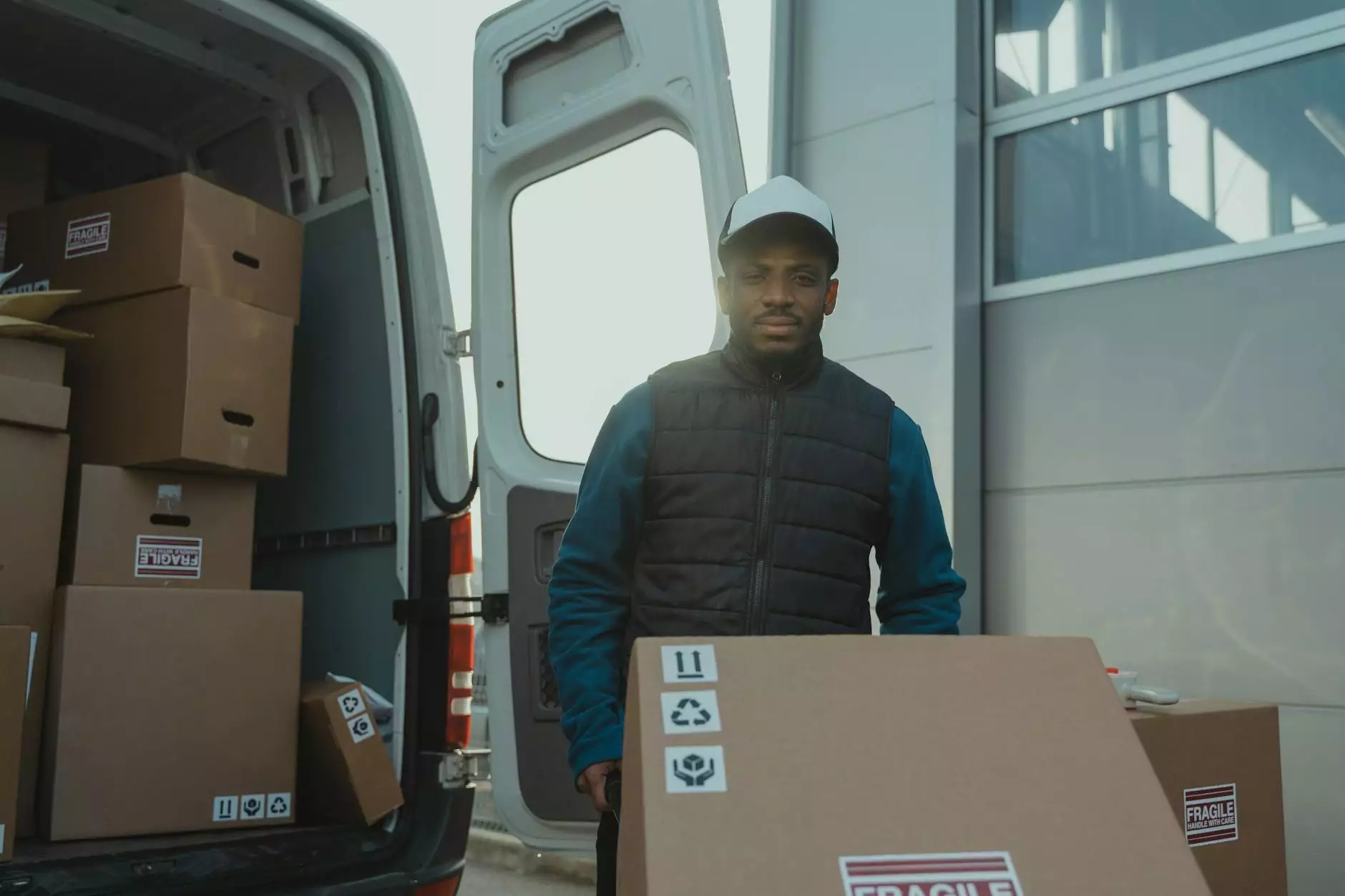
In today's rapidly evolving technological landscape, businesses are increasingly relying on artificial intelligence (AI) and machine learning (ML) to streamline operations, enhance customer experiences, and drive growth. A critical aspect of leveraging these technologies effectively is machine learning data labeling, a process that involves annotating data to train machine learning models. This article delves into the significance of data labeling, how it impacts various sectors including home services and locksmithing, and the best practices for implementing it in your business strategy.
What is Machine Learning Data Labeling?
Machine learning data labeling is the process of tagging data that will be used to train machine learning algorithms. This entails adding metadata to input data, which can be images, text, or audio. The labeled data serves as a foundation for the machine learning models to learn and make predictions based on new, unseen data. For instance, in a home service business that uses AI for customer interaction, labeling data correctly ensures that the AI can understand customer requests accurately and provide appropriate responses.
The Role of Data Labeling in Machine Learning
The efficacy of machine learning models is heavily reliant on the quality of the labeled data. Without accurate labels, the models cannot learn effectively, leading to poor performance in real-world applications. Here are some key roles that data labeling plays in machine learning:
- Training Data Creation: Labeled data is essential for training machine learning models, allowing them to learn patterns and make informed predictions.
- Quality Control: Proper data labeling ensures high-quality training sets, which reduces bias and errors in predictions.
- Model Evaluation: Labeled datasets are used to evaluate the performance of models by comparing predictions to actual outcomes.
- Feedback Loop: The process of labeling and re-labeling data creates a feedback loop that continually improves model accuracy.
Why Machine Learning Data Labeling Matters for Home Services
For businesses in the home services sector, such as locksmiths, accurate machine learning data labeling can be a game-changer:
Enhancing Customer Interaction
Incorporating AI into customer service chatbots can significantly enhance customer interaction. By training chatbots with accurately labeled historical conversation data, businesses can create models capable of understanding customer inquiries, predicting needs, and offering timely solutions — all while improving customer satisfaction.
Optimizing Service Delivery
Home service companies can leverage machine learning algorithms to analyze labeled data on past jobs, customer preferences, and service times. This analysis can drive better resource allocation, efficient scheduling, and even predictive maintenance, which ensures that clients receive timely assistance tailored to their needs.
Improving Marketing Strategies
Accurate data labeling enables home service providers to segment customers effectively and target them with personalized marketing campaigns. With labeled data from customer interactions and preferences, businesses can identify trends and develop strategies that resonate more with their target audience, increasing engagement and conversion rates.
Challenges in Machine Learning Data Labeling
While the benefits of machine learning data labeling are vast, there are numerous challenges that businesses must overcome:
- Data Diversity: Home service businesses often have varied customer demographics, requiring diverse data sets to capture different needs and preferences.
- Labeling Consistency: Achieving consistent labeling across multiple datasets can be challenging, especially when relying on multiple annotators.
- Scalability: As the business grows, the volume of data to be labeled increases, making it necessary to implement scalable labeling solutions.
- Quality Assurance: Ensuring high label quality can be resource-intensive, especially with large datasets; without quality assurance mechanisms, errors can propagate.
Best Practices for Effective Data Labeling
To harness the full potential of machine learning data labeling, businesses should implement the following best practices:
1. Define Clear Labeling Guidelines
Creating comprehensive guidelines helps labelers understand what constitutes each label, minimizing ambiguity and ensuring consistency across datasets.
2. Choose the Right Tools and Technologies
Investing in advanced data labeling tools can enhance efficiency, allowing for features such as automated suggestions and collaborative labeling environments.
3. Leverage Experienced Labelers
Utilizing experienced data annotators minimizes errors and increases the dataset's overall quality, ultimately leading to better model performance.
4. Implement Continuous Monitoring and Feedback
Establish a feedback loop where models are regularly updated with new labeled data, and labeling accuracy is evaluated frequently to adapt to changing requirements.
5. Collaborate with Data Scientists
Engaging with data scientists throughout the labeling process ensures that the data is being labeled in a way that benefits the model's training and future predictions.
Outsourcing Data Labeling: A Worthwhile Consideration
As the demand for labeled data increases, many businesses are considering outsourcing their labeling tasks. By partnering with specialized firms, businesses can:
- Access Expertise: Outsourcing provides access to professional labelers experienced in specific industries.
- Reduce Costs: It can be more economical than scaling an in-house team, allowing businesses to focus resources on core activities.
- Improve Efficiency: Specialized firms often have tools and processes in place that can streamline the labeling process.
The Future of Machine Learning Data Labeling
The future of machine learning data labeling looks bright as new technologies and methodologies continue to emerge. Innovations such as:
- Automated Labeling: Advances in AI are enabling systems to begin automatically labeling data, significantly reducing manual effort.
- Active Learning: This technique allows machine learning models to identify which data points are the most informative and should be labeled next, optimizing the data labeling process.
- Collaboration Platforms: New tools facilitate collaboration between labelers, data scientists, and machine learning engineers, making the process more efficient and integrated.
Conclusion
In conclusion, machine learning data labeling is an indispensable element of modern business practices, particularly within the home services and locksmithing sectors. By understanding the significance of data labeling and implementing best practices, businesses can leverage the power of AI effectively, driving efficiency, improving customer satisfaction, and fostering growth in an increasingly competitive landscape. Embracing the future of machine learning will not only keep businesses ahead of the curve but will also pave the way for innovative solutions that meet the dynamic needs of customers.